The event will take place virtually (on gather.town) on August 4-5 2021, during the virtual-only part of COLT 2021 conference. The event will have three components: academic workshop, technical talks and social events.
The academic workshop will focus on grad school admissions, fellowships as well as academic jobs.
The technical talks will feature 3 spotlight talks (15 minutes each) covering recent exciting work in learning theory.
The social component will include speed-networking, mentorship tables, games and more.
NEWS: WiML-T is organizing a career panel for Women in Learning Theory on the theme of "Career Path and Decisions in Industry and Academia" during the virtual-only part of COLT 2021. We highly recommend registering for the awesome event here.
Mentorship Workshop
Event Schedule
Welcome and Networking.
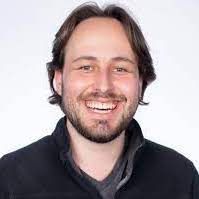
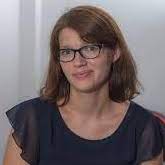
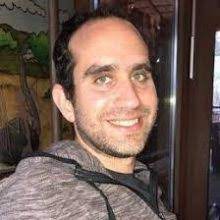
Spotlight Talks. Dylan Foster., Emilie Kaufmann., Shay Moran
Session chair: Manolis Zampetakis
Venue: Muenzinger Auditorium (gather.town)
See abstracts at the end of the schedule.
Break.
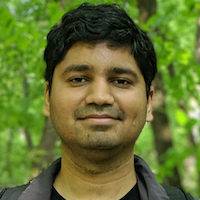
How-To Talk 1: Undergrad/Junior-grad. Pravesh Kothari (Parallel session) [Slides]
Session chair: Chara Podimata
Venue: Muenzinger Auditorium (gather.town)
This talk will cover general advice about applying to graduate schools and looking for fellowships.
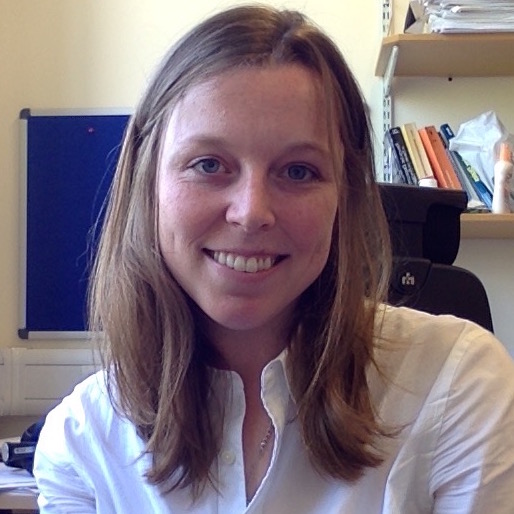
How-To Talk 1: Senior-grad/Postdoc. Cynthia Rush (Parallel session) [Slides]
Session chair: Cyril Zhang
Venue: Gold Auditorium (gather.town)
This talk will cover general advice about applying to academic jobs.
Break.
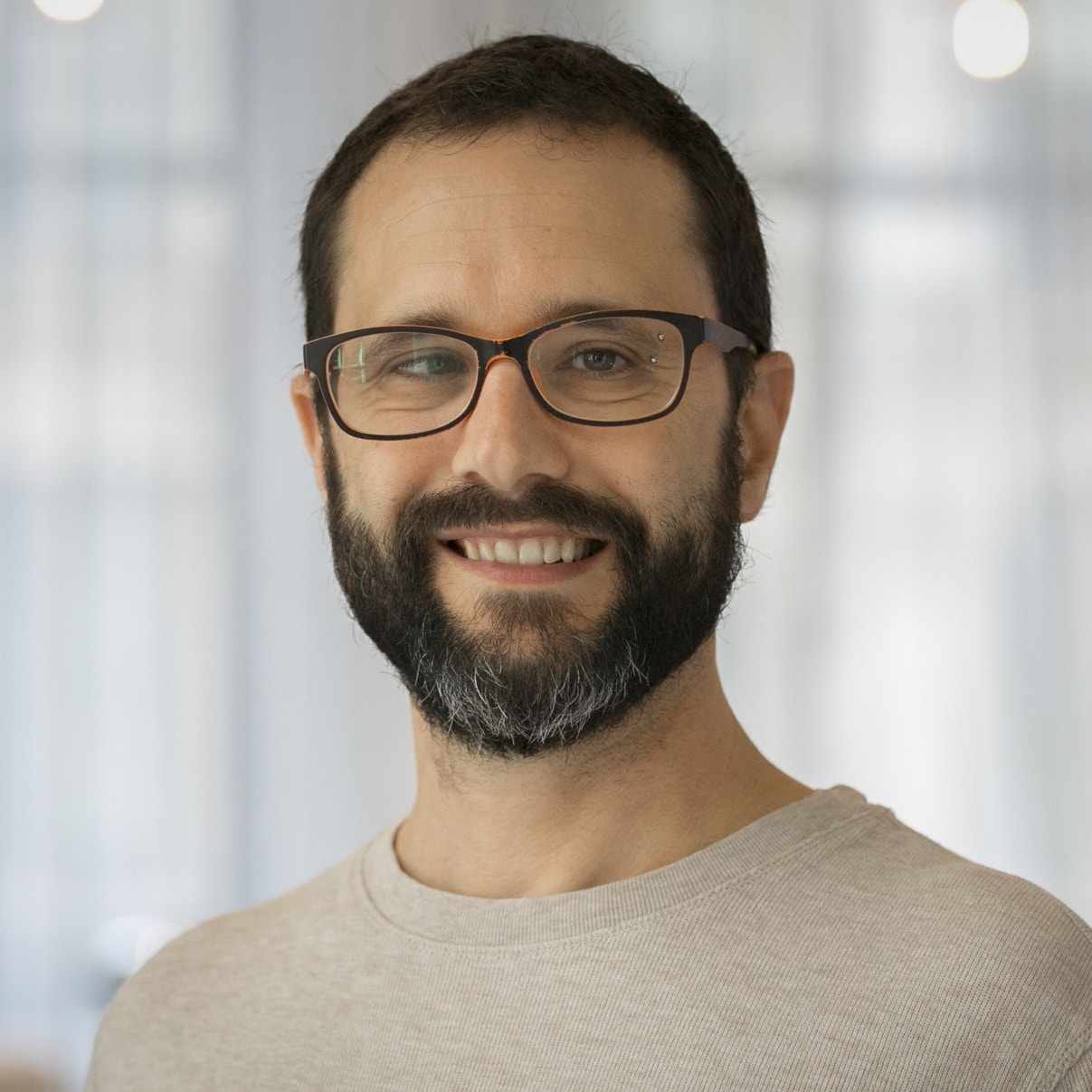
How-To Talk 2: Undergrad/Junior-grad. Boaz Barak (Parallel session) [Slides]
Session chair: Naama Ben David
Venue: Muenzinger Auditorium (gather.town)
This talk will cover how to write research statements and statements of purpose for grad school and fellowship applications. The talk will feature real statements written by members of the community which the speaker will dissect.
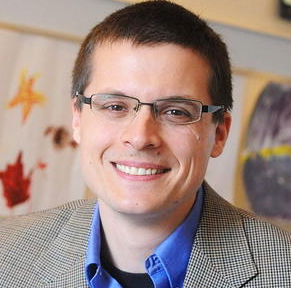
How-To Talk 2: Senior-grad/Postdoc. Rocco Servedio (Parallel session) [Slides]
Session chair: David Wajc
Venue: Gold Auditorium (gather.town)
This talk will cover how to write research statements for academic jobs. The talk will feature real statements written by members of the community which the speaker will dissect.
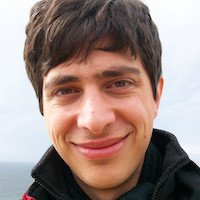
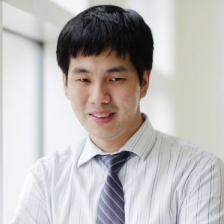
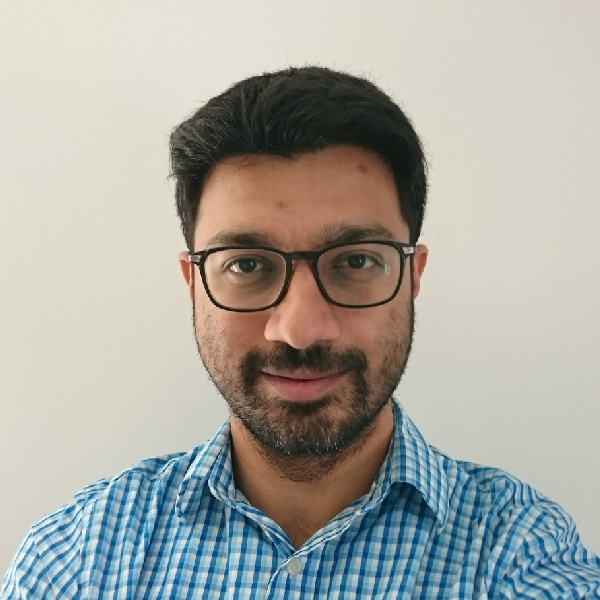
Panel Discussion: Undergrad/Junior-grad. Rafael Frongillo, Daniel Hsu, Satyen Kale (Parallel session)
Session chair: Ramya Korlakai Vinayak
Venue: Muenzinger Auditorium (gather.town)
This is a panel discussion about preparing applications for graduate schools and fellowships. Participants are encouraged to ask the panelists any questions on this topic.
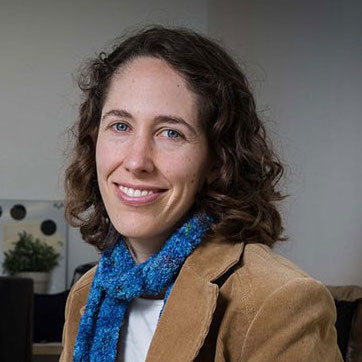
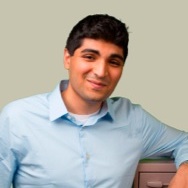
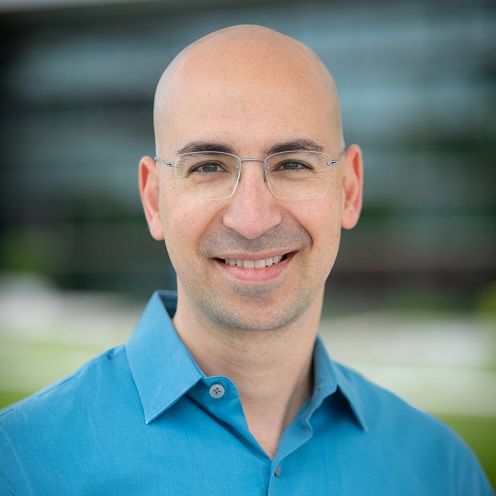
Panel Discussion: Senior-grad/Postdoc. Katrina Ligett, Ankur Moitra, Ariel Procaccia (Parallel session)
Session chair: John Wright
Venue: Gold Auditorium (gather.town)
This is a panel discussion about preparing applications for academic jobs. Participants are encouraged to ask the panelists any questions on this topic.
Meet & Greet.
This session is intended to be free time to socialize with senior members of the community as well as other participants. There will be tables where a senior member would be available to seek advice and answer your questions!
Spotlight Talk Abstracts.
- Bridging Learning and Decision Making: Recent Progress in Contextual Bandits. Dylan Foster. Machine learning is becoming widely used in decision making, in domains ranging from personalized medicine and mobile health to online education and recommendation systems. While (supervised) machine learning traditionally excels at prediction problems, decision making requires answering questions that are counterfactual in nature, and ignoring this mismatch leads to unreliable decisions. As a consequence, our understanding of the algorithmic foundations for data-driven decision making is limited, and efficient algorithms are typically developed on an ad hoc basis. Can we bridge this gap and make decision making as easy as machine learning? Focusing on the contextual bandit, a core problem in data-driven decision making, we bridge the gap by providing the first optimal and efficient reduction to supervised machine learning. The algorithm allows users to seamlessly apply off-the-shelf supervised learning models and methods to make decisions on the fly, and has been implemented in widely-used, industry-standard tools for decision making.
- Non-parametric exploration in multi-armed bandits. Emilie Kaufmann. Multi-Armed Bandit models are powerful to describe several sequential resource allocation tasks in a stochastic environments, e.g., the design of a recommendation algorithm or an adaptive clinical trial. This simple model also captures the exploration/exploitation dilemma that is central in more structured reinforcement learning problems. The two most famous approaches to MABs, namely Upper Confidence Bounds and Thompson Sampling, share the need for some prior information about the arms’ distributions in order to attain optimal performance. We will discuss other families of algorithms based on sub-sampling that perform well in practice and can be proved to be optimal for different families of distributions.
- Boosting Simple Learners. Shay Moran Boosting is a celebrated machine learning approach which is based on the idea of combining weak and moderately inaccurate hypotheses to a strong and accurate one. We study boosting under the assumption that the weak hypotheses belong to a class of bounded capacity. This assumption is inspired by the common convention that weak hypotheses are “rules-of-thumbs” from an “easy-to-learn class”. (Schapire and Freund ’12, Shalev-Shwartz and Ben-David ’14.) Formally, we assume the class of weak hypotheses has a bounded VC dimension. We focus on two main questions:
- Oracle Complexity: How many weak hypotheses are needed in order to produce an accurate hypothesis? We design a novel boosting algorithm and demonstrate that it circumvents a classical lower bound by Freund and Schapire (’95, ’12). Unlike previous boosting algorithms which aggregate the weak hypotheses by majority votes, the new boosting algorithm uses more complex (“deeper”) aggregation rules. We complement this result by showing that complex aggregation rules are in fact necessary to circumvent the aforementioned lower bound.
- Expressivity: Which tasks can be learned by boosting weak hypotheses from a bounded VC class? Can complex concepts that are “far away” from the class be learned? Towards answering the first question we identify a combinatorial-geometric parameter which captures the expressivity of base-classes in boosting. Along the way, we establish and exploit connections with Discrepancy Theory.
Welcome and Networking.
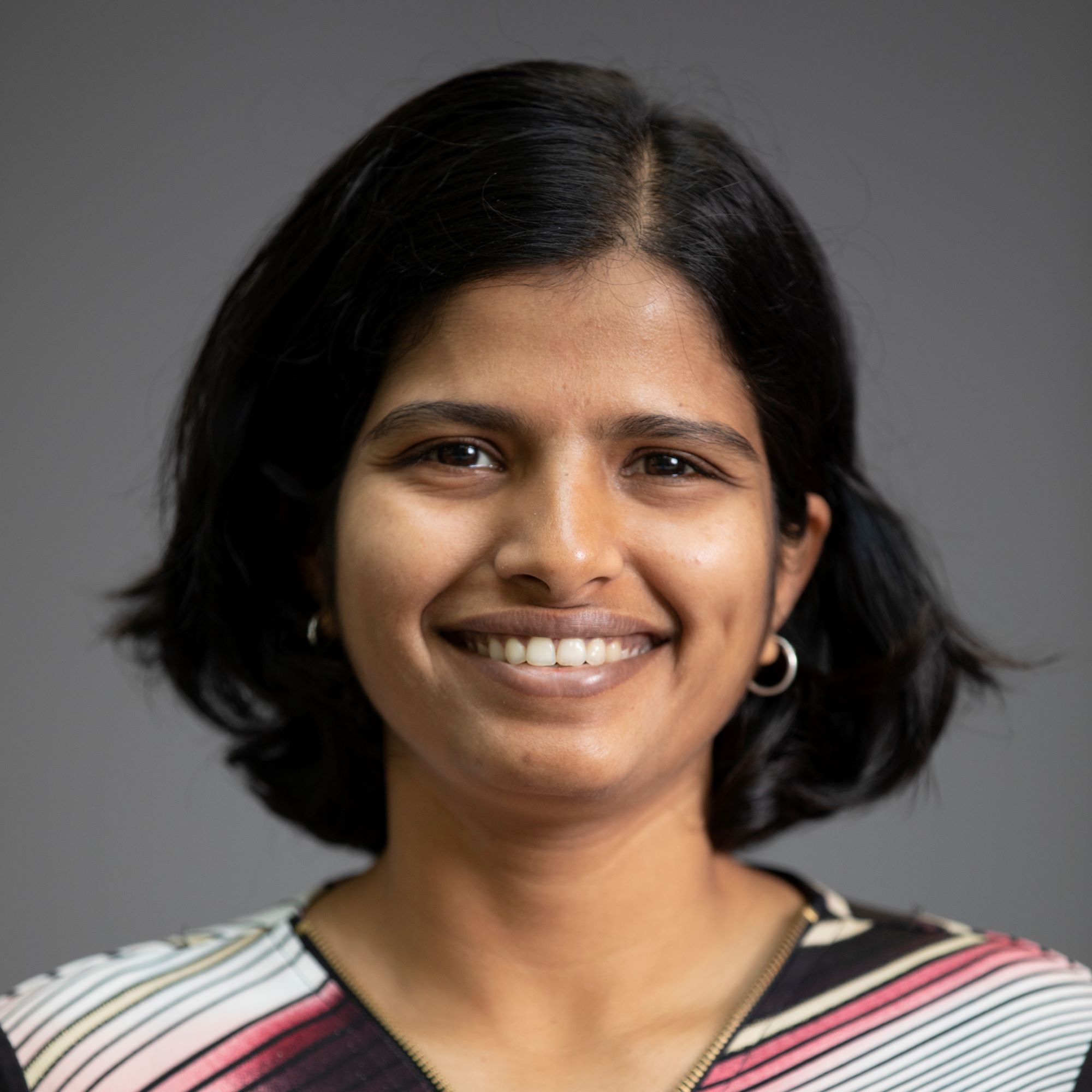
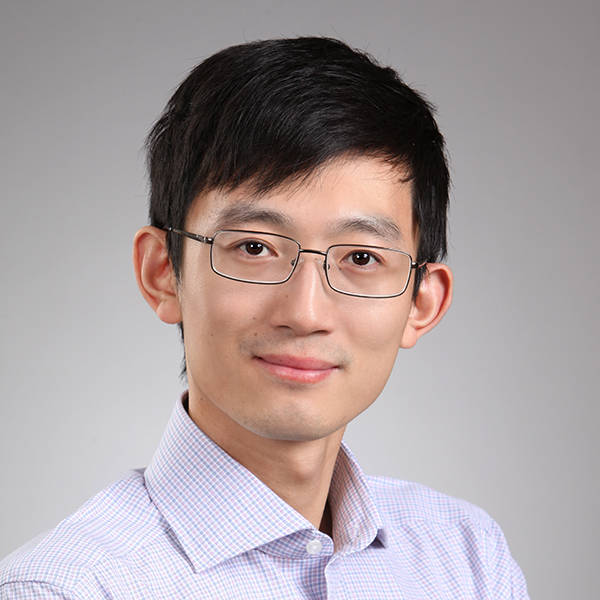
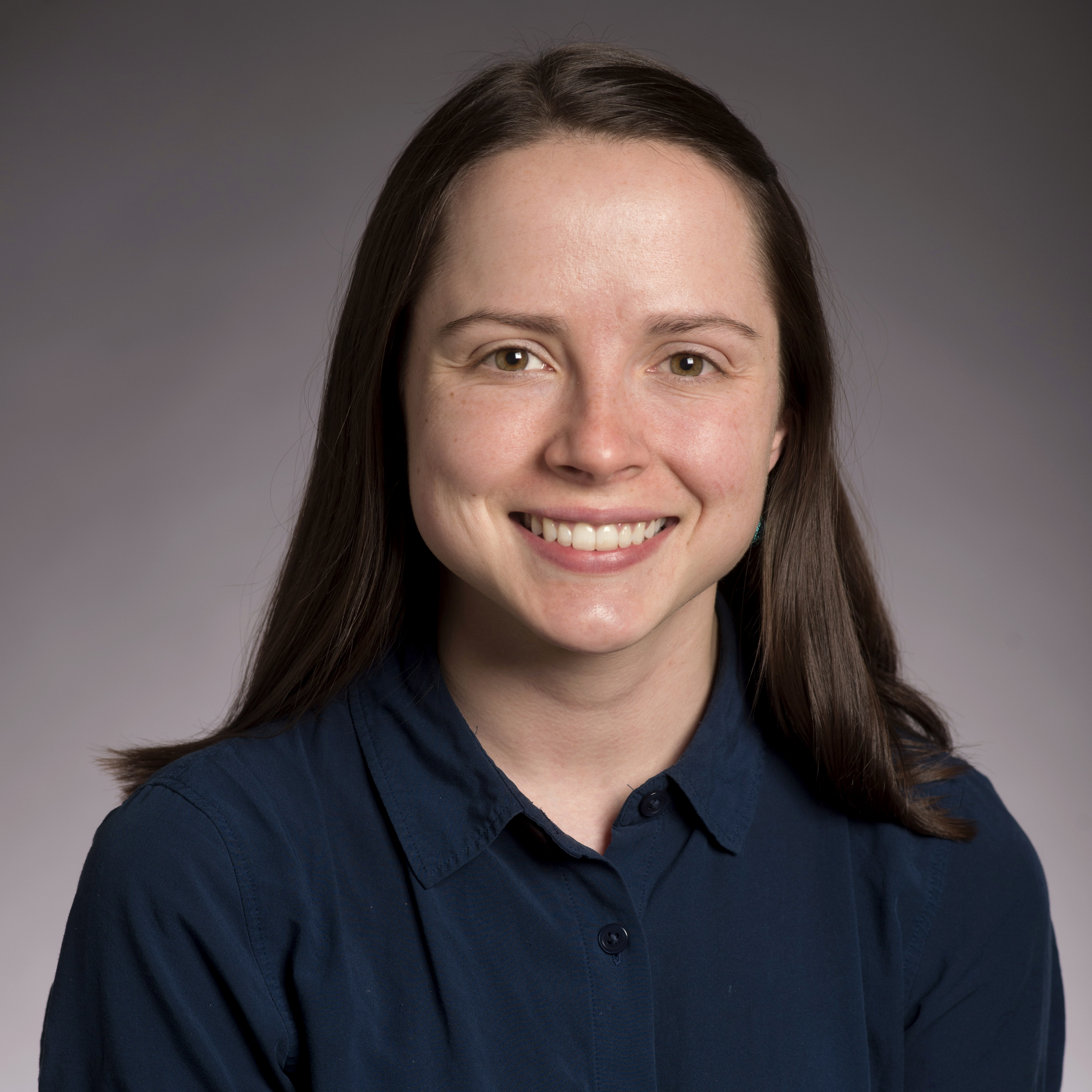
Spotlight Talks. Suriya Gunasekar., Tengyu Ma, Ellen Vitercik.
Session chair: Thodoris Lykouris
Venue: Muenzinger Auditorium (gather.town)
See abstracts at the end of the schedule.
Break.
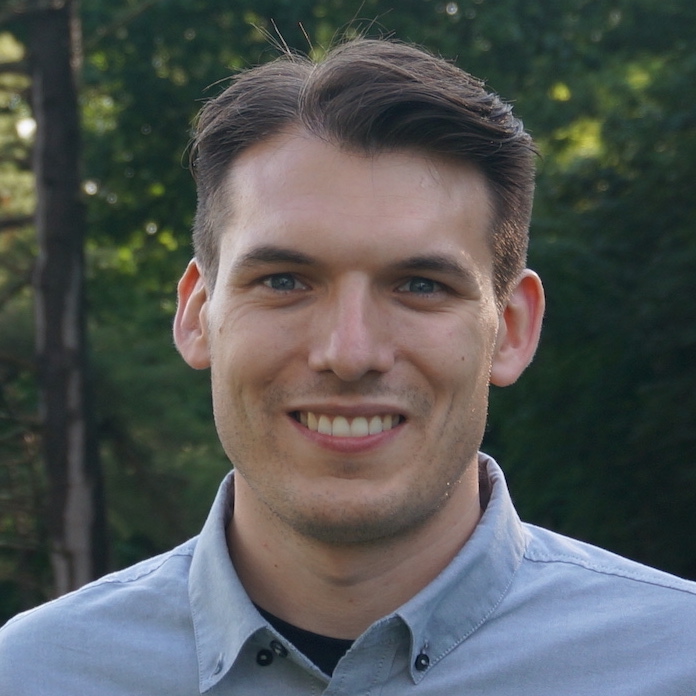
How-To Talk 1: Undergrad/Junior-grad. Kevin Jamieson [Slides]
Venue: Muenzinger Auditorium (gather.town)
This talk will cover general advice about applying to graduate schools and looking for fellowships.
Break.
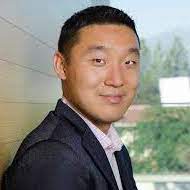
How-To Talk 2: Undergrad/Junior-grad. Yisong Yue [Slides]
Venue: Muenzinger Auditorium (gather.town)
This talk will cover how to write research statements and statements of purpose for grad school and fellowship applications. The talk will feature real statements written by members of the community which the speaker will dissect.
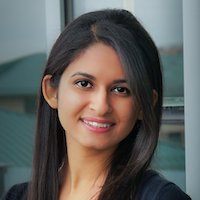
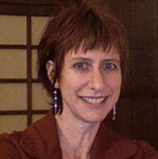
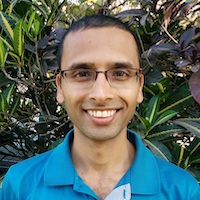
Panel Discussion: Undergrad/Junior-grad. Nika Haghtalab, Anna Karlin, Praneeth Netrapalli
Venue: Muenzinger Auditorium (gather.town)
This is a panel discussion about preparing applications for graduate schools and fellowships. Participants are encouraged to ask the panelists any questions on this topic.
Meet & Greet.
This session is intended to be free time to socialize with senior members of the community as well as other participants. There will be tables where a senior member would be available to seek advice and answer your questions!
Spotlight Talk Abstracts.
- Implicit regularization from optimization algorithms in learning. Suriya Gunasekar. Understanding how optimization algorithms implicitly control capacity of overparametrized models is an active area of research. In this talk, I will overview some results illustrating this phenomenon and enumerate a few promising directions for future research.
- Provable Guarantees for Self-Supervised Deep Learning with Spectral Contrastive Loss. Tengyu Ma. Recent works in self-supervised learning have advanced the state-of-the-art by relying on the contrastive learning paradigm, which learns representations by pushing positive pairs, or similar examples from the same class, closer together while keeping negative pairs far apart. Despite the empirical successes, theoretical foundations are limited -- prior analyses assume conditional independence of the positive pairs given the same class label, but recent empirical applications use heavily correlated positive pairs (i.e., data augmentations of the same image). In this talk, I will present a new work that analyzes contrastive learning without assuming conditional independence of positive pairs using a novel concept of the augmentation graph on data. Based on joint work with Jeff Z. Haochen, Colin Wei, Adrien Gaidon: [paper].
- How much data is sufficient to learn high-performing algorithms? Ellen Vitercik. Algorithms often have tunable parameters that have a considerable impact on their runtime and solution quality. A growing body of research has demonstrated that data-driven algorithm design can lead to significant gains in runtime and solution quality. Data-driven algorithm design uses a training set of problem instances sampled from an unknown, application-specific distribution and returns a parameter setting with strong average performance on the training set. We provide a broadly applicable theory for deriving generalization guarantees for data-driven algorithm design, which bound the difference between the algorithm's expected performance and its average performance over the training set. The challenge is that for many combinatorial algorithms, performance is a volatile function of the parameters: slightly perturbing the parameters can cause a cascade of changes in the algorithm’s behavior. Prior research has proved generalization bounds by employing case-by-case analyses of parameterized greedy algorithms, clustering algorithms, integer programming algorithms, and selling mechanisms. We uncover a unifying structure which we use to prove very general guarantees, yet we recover the bounds from prior research. Our guarantees apply whenever an algorithm's performance is a piecewise-constant, -linear, or—more generally—piecewise-structured function of its parameters. As we demonstrate, our theory also implies novel bounds for dynamic programming algorithms used in computational biology and voting mechanisms from economics. This talk is based on joint work with Nina Balcan, Dan DeBlasio, Travis Dick, Carl Kingsford, and Tuomas Sandholm.